Algorithms Ruling the Market: Can AI Be Trusted with Your Investments?
- Arda Keskinpala
- Oct 28, 2024
- 6 min read
Updated: Nov 2, 2024
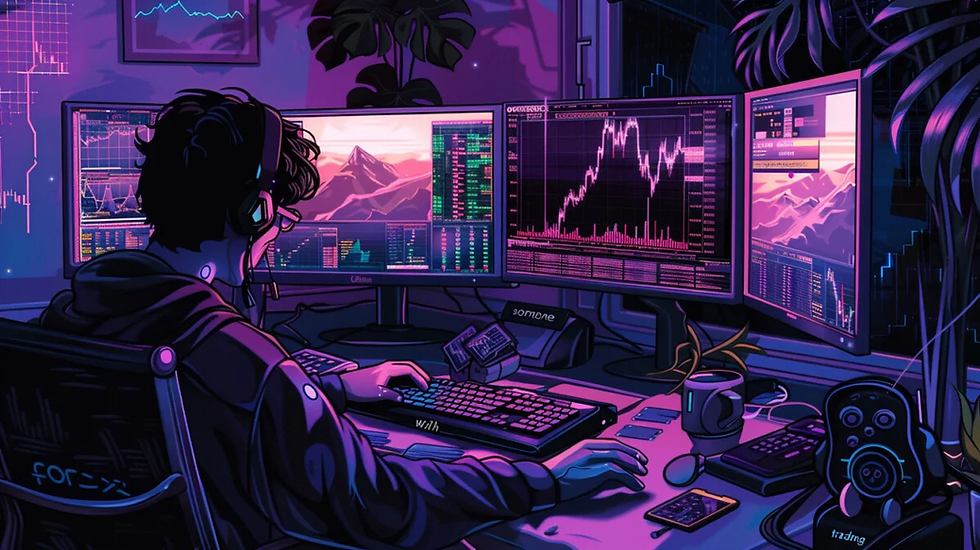
By: Arda Keskinpala
The stock market, previously long driven by human analysis, decision making, and risk assessment, has been experiencing a revolution. Artificial intelligence as we must know by now, has been on the rise, technologies including machine learning, natural language processing, and high-frequency trading algorithms have been reshaping how investors approach the stock market and finance in general. In fact, a recent Gartner survey of 121 finance leaders has revealed that 58% of organizations are using financial AI in 2024, which is a 34% increase from the previous year. This rapid increase highlights how AI has become a fundamental aspect of modern financial markets – from stock price prediction to real-time trading decisions. While AI’s offers on investors seems immense for maximizing efficiency and profitability, it also brings forth conversations about the danger of market manipulations, fairness, and transparency.
The Evolution of AI in Stock Trading
The introduction of AI in stock trading stems from the concept of algorithmic trading which traces back to the early 1990s, where the first algorithmic trading systems were developed. These systems were designed to minimize the nature of human intervention in manual trading, in order to avoid the errors that are present due to human decisions. In order to do so, they automated the process that manual investors would originally do- they automatically generated and executed trades in the stock market to maximize success. They analyzed past and current market data to identify buying/selling opportunities, which could be modified to agree to different criteria.
Enhancing the backbone of algorithmic trading automation, the integration of machine learning (ML) into the stock market became a significant shift from the early days of algorithmic trading. ML introduced the ability for AI to learn from data and improve over time, adapting as more data became available. These models became increasingly valuable as they leveraged historical stock prices & events, technical indicators, and data to make accurate predictions about future movements in the market.
In addition, these AI models began to integrate sentiment analysis – the process of computationally identifying and categorizing opinions expressed in text, in order to determine the writer’s attitude towards a topic – with the utilization of natural language processing (NLP). When combined, these algorithms can gauge sentiment from various sources such as news articles, social media, and other trusted sources. This brought forth predictive analysis on the fluctuation of the market, helping to anticipate stock movements potentially influenced by events or public sentiment.
With the incorporation of ML and sentimental analysis emerged predictive analysis – where AI could anticipate fluctuations in the market driven by factors such as company earnings announcements, political developments, and social reactions on social media regarding events.
High Frequency Tarding (HFT) and AI
Becoming prominent in the early-middle 2000s, high-frequency trading (HFT) revolutionized the trading environment – HFT is a trading method which utilizes powerful computer programs to transact a large number of orders in a fraction of a second. In the context of financial AI, HFT uses complex algorithms in order to analyze multiple markets, and execute orders correspondent to the market conditions analyzed. In terms of financial AI in correspondence to HFT contributes to the improvement of market liquidity. This is due to the mass volume of trades that are being executed in a minimal amount of time, which increases the number of buy-and-sell orders in the market. This liquidity is essential for market stability, and ensures that investors have freedom in their decisions.
As high-frequency trading has its benefits regarding the ease and speed at which transactions can be executed, it consequently accompanies controversial opinions, and harsh criticism. Due to its abundance of models based on mathematics, it flushes out human interaction and decision out of the deal. Due to the sole speed of decisions made by these AI, they have the grave potential to result in major market volatility without reason. An example of this includes the Dow Jones Industrial Average flash crash in 2010, where they suffered a loss of 10% in 20 minutes before rising again. However, proponents still believe that HFT’s provide a profound sense of liquidity and efficiency to the stock market.
AI-Driven Hedge Funds
AI-driven hedge funds – or quantitative funds (quant funds), have transitioned to a significant force in finance. Quant funds are investment funds, and they rely heavily on AI models to make decisions regarding investments by leveraging algorithms, machine learning, to pin-point profitable opportunities. As opposed to hedge funds and human managers, quant funds lack the parameters of judgement, experience, and opinions in the process of making investment decisions. Quant funds have been fueled due to the greater access of market data, allowing them to conduct broader analysis on greater scenarios and time horizons making predictions increasingly accurate.
The success of these quantitative funds heavily relies on the vast array of trading signals that they utilize. These range from economic data points, to trending global asset values and company news. They have been favorable due to the number of returns that they have generated, however have fallen-off, and have been underperforming since 2016 – as stated by a report by Institutional Investor.
However, these AI-powered hedge funds have also demonstrated success, outperforming original investment strategies. One example of this is the Renaissance Technologies, which consistently had high returns by employing AI models to make high-speed decisions, with a basis from data – they had an average annual return of 40% net over more than three decades. As seen, these types of hedge funds have massive capabilities for returns due to their ability to adapt quickly to changing market conditions, due to the analysis through methods previously mentioned.
Market Manipulation Concerns
As mentioned, and as we have seen, AI has become a core fragment integral to stock trading. However, with such immense potential and value, comes the concerns. Market manipulation and fairness has emerged from these AI-powered trading systems. Regardless of the mass benefits of AI in the market, the detrimental potential to manipulate markets/create unfair advantages has raised ethical and regulatory challenges regarding this matter.
One of the main concerns is that when AI-driven trading is coupled with HFT firms or hedge funds, it can execute trades at speeds too quick for regular traders, which can hamper regular stock prices and volatility. These systems that are so powerful can exploit price discrepancies – identified in milliseconds – causing sudden market shifts. The big-picture concern as a result of this is that it creates a disparity between firms with advanced AI systems and with traditional investors.
One prominent and recent example of this is the GameStop short squeeze in January of 2021, where a group of individual retail investors organized mass purchase of GameStop stock with the price skyrocketing as a result. GameStop stock had gone from a previous price of $20 in January, to greater than $400 at its peak. What these users buying frenzy did was squeeze AI-driven hedge funds that had heavily shorted GameStop. In essence, this situation demonstrates how market manipulation can happen as a result of a group of retail traders coordinating to interact with AI and algorithmic systems to emerge unusual market behavior.
Future Trends and Developments
As AI continues to advance, the influence that it may have on the stock market will be vastly greater. Some key developments that may occur include:
- More accurate Predictions – As AI models become better at analyzing complex data, they will consequently produce better market predictions.
- Autonomous Trading – Fully automated trading systems might become normalized, with decreasing necessity for human oversight.
- Personalized Investing – Retail investors might have access to AI tools allowing for them to tailor portfolio management, which might level the unfairness between big corporations and individual traders.
- Stronger Regulations - There might be a push for more robust regulations to ensure fairness, and methods of avoiding mass retail market manipulation.
Conclusion
As AI is reshaping the stock market, it offers both great opportunities but with consequent challenges. The future of AI is promising for improved efficiency and profitability through the stock market. However, one thing to think about is that as technology evolves, it may be harder to keep up with the promotion of the fairness, transparency, and integrity of financial markets.
References
Malz, A. (2022). The GameStop Episode: What Happened and What Does It Mean? Cato.org. https://www.cato.org/cato-journal/fall-2021/gamestop-episode-what-happened-what-does-it-mean
Quant Fund: Definition, How They Work, Performance, and Risks. (n.d.). Investopedia. https://www.investopedia.com/terms/q/quantfund.asp
mids.capital. (2024, January 24). Evolution of Algorithmic Trading: Past, Present, and Future Trends. Medium. https://midscapital.medium.com/evolution-of-algorithmic-trading-past-present-and-future-trends-46fd9680b0da
Machine Learning in Algorithmic Trading. (n.d.). Deep gram. https://deepgram.com/ai-glossary/machine-learning-algorithmic-trading
IBM. (2023). What is sentiment analysis? | IBM. Www.ibm.com. https://www.ibm.com/topics/sentiment-analysis
Davies, A. (2022, August 21). How to Use Machine Learning in Algorithmic Trading? - DevTeam.Space. DevTeam.Space. https://www.devteam.space/blog/machine-learning-for-algorithmic-trading/
Algomojo. (n.d.). Evolution of Algorithmic Trading – Algo Insights. https://algomojo.com/blog/evolution-of-algorithmic-trading/
Strickland, B. (2024, September 16). More organizations are turning to AI in the finance function. Journal of Accountancy; Journal of Accountancy. https://www.journalofaccountancy.com/news/2024/sep/organizations-turning-to-ai-in-finance-function.html#:~:text=Adoption%20of%20finance%20AI%20%E2%80%94%20artificial
Comments